Automous Machines — Sci-fi becomes Science Fact

Autonomous Machines — Sci-fi becomes Science Fact
When I was young, one of the scariest films I watched was Westworld, and where Yul Bruner played the robotic gunslinger who just would give up. In the film, a software bug drove “him” to misfunction. But these days, could you also add a cyber or ransomware attack?
Well, imagine a world where autonomous machines decided that they wanted to go out in the evening rather than being constrained by their owners, and where robots vehicles cleaned the streets and picked up refuge. With the power of computers, the reality of machines not obeying their “owners” is now with us, and we must understand the risks that these pose to our society.
In a classic Red Dwarf, even a toaster had its own personality:
In another episode, the vending machines decided to go on strike, as they wanted to do something other than their given roles.
The rise of the autonomous and intelligent machine projects a work where machines can reason and make their own decisions. At the far end of the spectrum is where a machine either makes the wrong decisions at risks lives or actually decides to kill, in order to achieve its mission. While it sounds like science-fiction, it is in fact a reality, and where a car designer must decide whether their mission is to protect the person in the car or those outside it.
And so on 30 June 2021, it was reported that a Tesla car would found to be on fire after driving around on its own:

But imagines that a robot killer is on the loose, and “it” is moving through a marketplace in search of its human target. It fitted with the sensors required to look for human life, with radars, infrared transmitters and sensors, and stereoscopic vision. The killer monitors the heat from humans and fires a sprinkle of infrared dots onto the faces of the humans it senses, and stores these as 3D maps, and marks for further processing. It monitors its own position using GPS and landmarks, and maps these onto a map of the area — continually adding new objects that it sees along the way. But, it only has one mission, and that is to fire its weapon into the heart of its target.
Along the way it scans faces and records match to their gender, their expressions, their age, and if it can, their names. Each data point is recorded, and classified, and fed into its running program. It has a mission, but it learns new things as it goes. Our robot senses that it is nearing the area of its target and moves into kill mode. All of its processing is now focused on finding the target. Audio and video sensors are now set into hi-resolution mode, and all of its processing is focused on finding a match for its target.
But, there’s a big bang, as a champagne cork pops from a bottle. This is nothing that the robot has ever heard before. It scans the area for the source and tries to find a match to something that it has heard before or in its data bank of sounds. Its best match is “gunfire”. In its training up to now, gunfire was always a sign of trouble, and, so, it must preserve itself. The robot turns and runs from the area. And as it runs, it feels the fear of an attack. It senses the area for weapons and adversaries, and must now find a place of safety. It has been in this situation before in the lab and must preserve itself. The mission is just too important for it to be harmed. It must flee away from danger, and return at a later time than to stay and risk being destroyed. It has learnt this from its “playtime” in the lab, and now it is being enacted for real. Self-preservation becomes key to the delivery of the mission.
While this may seem like it is something from a science fiction film, the rise of autonomous systems may see this type of scenario coming true in the near future. For years we have seen computers beat humans in areas that humans were always better, but in the next decade or so will them learning independently from us, and in making sense of an uncertain world.
From traditional warfare to AI warfare
Many believe that cyber warfare and AI will replace our existing methods of war. And so it is perhaps not “if” … but “when” … cyber warfare will happen. It could also signal (or trigger) the first phases of warfare between two countries. Unfortunately, when the NATO Treaty was signed, there was no such thing as a cyber attack, and many countries are now debating whether a cyberattack could constitute an act of war. One must thus worry that there are places in the world where there is already tension, a perceived attack could trigger warfare.
So, while cyberespionage is commonplace, the legal system of most countries has not crystallized on the concept of cyber warfare and in the usage of AI in traditional ways of warfare. With cyber espionage, though, there is no physical damage and it does not do any physical harm, but cyber warfare could cost many lives. Many worry, too, that a perceived cyber-attack from a rogue group could be mistaken for a nation-state attack, and then trigger a war between countries.
One model of warfare for the future would be to conduct cyber warfare to disrupt and decimate the infrastructure of a country, and then followed up with killer robots, and which could flood into a country and take ultimate control. Whereas warfare of the past waged wars that lasted for decades, the future may be towards wars that could be launched, executed and completed within hours. The attack itself could perhaps not involve a single person on the attacking side losing their lives, but lead to large scale devastation of the targeted country. Many see this as a war of machines and algorithms.
Machines v Humans
In 1950, Alan Turing declared that one day computers will have the same intelligence as humans, and proved it with a special test. For this, he asked human testers to ask a human and a computer random questions. If the computer gave the correct answer, the testers could not differentiate between the human and the computer.
At present, computers and humans each have advantages over each other, but as computers become faster and contain more memory, they can replace humans in many situations. In Arthur C.Clarke’s 2001: A Space Odyssey, the spacecraft’s on-board computer, HAL, played the captain at chess. The computer won and then took over the ship. Thus, if a computer could beat the best human intellect at a game which provided one of the greatest human challenges then they are certainly capable of taking on the most complex of problems. This became a reality when, on 10th February 1996, Deep Blue, a computer developed by IBM, beat Gary Kasparov at chess in a match in the USA. It was a triumph for AI.

Gary actually went on to beat Deep Blue by four games to two, but the damage had already been done. It would only be a matter of time before a computer would beat the chess champion, as, on average, they increase their processing capacity each year, as well as improving their operation and the amount of information they can store. Thus, in a rematch in May 1997, Deeper Blue, the big brother of Deep Blue, beat Gary by 3½ to 2½. The best computer had finally beaten the best human brain. In reality, it was an unfair challenge. Computers have a massive number of openings programmed into it, and can search through an almost infinite amount of situations. Kasparov knew the only way to beat the computer was to get it away from its opening encyclopedia as quickly as possible, he thus made moves which would be perceived as bad when playing against another human. An annoying feature of playing against a computer is that it never makes mistakes, as humans do. Computers also process data faster than the human brain and can search billions of different options to find the best.
Claude Shannon, in 1949, listed the levels in which computers could operate, each with a higher level of intellectual operation. Figure 1 outlines these. Fortunately humans have several advantages over computers. These are:
- Learning. Humans adapt to changing situations, and generally quickly learn tasks. Unfortunately, once these tasks have been learnt, they often lead to boredom if they are repeated repetitively.
- Strategy. Humans are excellent at taking complex tasks and splitting them into smaller, less complex, tasks. Then, knowing the outcome, they can implement these in the required way but can make changes depending on conditions.
- Enterprise. Computers, as they are programmed at the present, are an excellent business tool. They generally allow better decision making, but, at present, they cannot initiate new events.
- Creativity. As with enterprise, humans are generally more creative than computers. This will change over the coming years as they programmed with the aid of psychologists, musicians and artists, and will contain elements that are pleasing to the human senses.

There are seven main components of human intelligence, which, if computers are to match humans, they must implement:
- Spatial. This is basically the ability to differentiate between two- and three-dimensional objects. Computers, even running power image processing software, often have difficulty in differentiating between a two-dimensional object and a three-dimensional object. Humans find this easy, and are only tricked by optical illusions, where a two-dimensional object is actually a three-dimensional object, and so on. The objects on the right-hand side are a mixture of two-dimensional and three-dimensional objects. Humans can quickly determine from the simple sketches that the top two objects are three-dimensional objects, which have been drawn as a two-dimensional object, and the lower one is either a two-dimensional object, or that it is a three-dimensional object that has been drawn from above. A computer would not be able to make these observations, as it would not understand how these sketches relate to real-life objects, and that they were actually three-dimensional objects.
- Perception. This is the skill of identifying simple shapes from complex ones. For example, humans can quickly look at a picture and determine the repeated sequences, shapes, and so on. For example, look at the picture on the right-hand side, and determine how many triangles that it contains. It is relatively easy for a human to determine this, as they have great perception skills. This is because the human brain can easily find simple shapes from complex ones. Imagine writing a computer program that would determine all of the triangles in an object, then modifying it so it finds other shapes, such as squares, hexagons, and so on.
- Memory. This is the skill of memorizing and recalling objects which do not have any logical connection. Humans have an amazing capacity for recalling previous objects, typically by linking objects, from one to the next. Computers can implement this with a linked-list approach, but it becomes almost impossible to manage when the number of objects becomes large.
- Numerical. Most humans can manipulate numbers in various ways. Humans are by no way as fast as computers, but humans can often simply complex calculations, but approximating, or by eliminating terms that have little effect on the final answer. For example: What is the approximate area for a room that is 6.9 metres and 9.1 meters? [Many humans would approximate this to 7 times 9, and say that it is approximately 63 meters squared, whereas a calculator would say 62.79 meters squared]
- Verbal. This is the comprehension of language. Many computers now have the processing capability to speech in a near-human form. It is also now possible to even computer computers accents, but it will be a long time before computers automatically learning a verbal language without requiring the user to train them.
- Lexical. This is a manipulation of vocabulary. Why is it that Winnie the Pooh more interesting to read than a Soccer match report? It’s all down to lexical skill. Currently, virtually all of the creative writing in the world has been created by humans, as humans understand how sentences can be made from a collection of words, and in a form which is interesting for someone to read. Computers are very good at spotting spelling mistakes, and even at finding grammatical errors, but they are not so good at actually writing the material in the first place. When was the last time that you read something that was originated from a computer? Have a look at the text in the box on the right-hand side of this paragraph. One of the sentences is active and interesting, while the other is passive and dull. Writing which is passive becomes boring to read, and most readers lose interest in reading it.
- Reasoning. This is induction and deduction. Humans can often deduce things when they are not given a complete set of information.
Cummings [2] outlines the areas in which machines are better than humans, and vice versa (Table 1). Overall machines are better than humans at speed, consistency, deductive reasoning, repetition, and sensing qualitative information, but humans are generally better at inductive reasoning, pattern recognition and variation in a task. Machines are also good at recording literal versions of data, while humans map memory into principles and strategies. This type of strength make humans better at innovation and are generally more versatile in quickly learning new tasks. With existing machine learning models, a car-building robot fits into a traditional model of an intelligent machine, but new cognitive models could allow machines to take over from humans in the areas that they generally are stronger in. Once a machine can think and learn on its own, we will see AI challenging our simple models of machine learning.

The cognitive abilities of machines have improved over the years, especially in prioritizing key incoming alerts. Humans are generally good at spotting a significant alert and filter other distractions out and thus focus on coping with it. A human controller within an industrial control system will often be expert at spotting an alarm which is the most significant and then filter out other alarms which have been triggered. If there are thus many alerts that can trigger, it would often be too complex to use a knowledge-based approach and to map all of the possible combinations through a rules-based approach. A cognitive model could thus be built over time, and start to define the required actions.
A skill-based task is thus often easy to replicate with a rule-based approach, but more complex tasks — such as flying an airplane in an emergency situation — require judgment and intuition and these normally require a cognitive model of learning. A true expert can thus react quickly to an uncertain event. It is the task of cognitive machine learning to match humans in becoming experts in making good decisions within complex and ever-changing tasks.
From autonomous UAVs to autonomous vehicles
With an autonomous UAV (Unmanned Aerial Vehicles), we can map the world into flying routes, obstacles and areas which should be avoided. Radar and GPS can then map around the complexity of the fly zone. With an automated car, we have a more complex environment and where we now need to add objects — such as pedestrians and other cars. These must be identified in real-time, and then tracked.
A vehicle must then predict objects around itself, and then predict where these objects will next move. This will then be used to make a decision on the movement of the vehicle, in order to complete its mission: to protect the driver of the vehicle, other humans, and other vehicles, and then to arrive at a destination. For this, we need LIDAR (Light Detection And Ranging — and which is commonly used for object mapping), radar and stereo vision cameras (Figure 2). Increasingly, too, self-driving vehicles use infrared scanning for the detection of human bodies and GPS sensing to map to known geographical maps of an area. A failure in any of the sensors must be coped with, and where the machine has to make decisions in challenging situations.
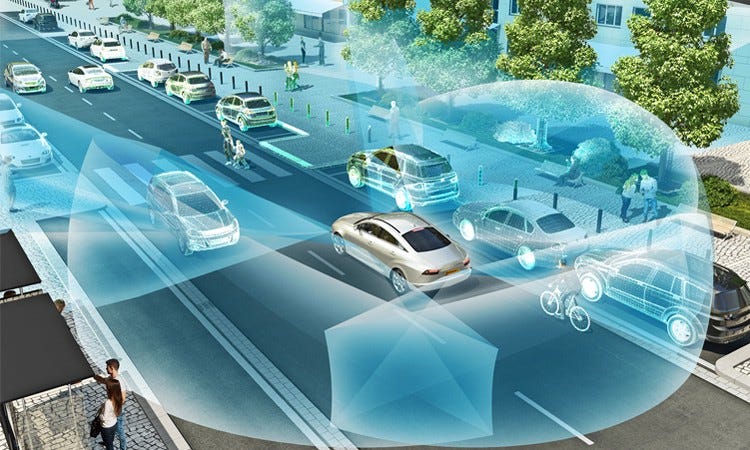
While predicting where objects will go, is a relatively easy task for a human, a computer needs a great deal of computing power to analyze the probabilities involved. The vehicle thus takes guesses and defines confidence intervals:
“Based on previous movements, there is a 50% chance that Pedestrian A will move into the path of our vehicle within the next three seconds, and a 30% chance that they will stop, and a 20% chance that they will move away from the path of the vehicle”.
This calculation must be continually computed, along with the path of the vehicle. The balance of power between the human and the machine will then be a decision on whether the machine can cope with every environment it is faced with. While the ethical issues around self-driving cars are clear, the usage of autonomous vehicles in military operations is less clear.
Robots and Warfare
The usage of AI in warfare will come from many angles includes the usage of robots to conduct missions against an enemy. While robots can be made robust than humans on the battlefield, they may also be able to have extra abilities in focused activities. This could include the finding and detonation of bombs. But their use up to now has not included dealing with complex situations, especially within a chaotic environment. The growth in driverless cars and drones shows that AI could be used in a warfare environment, especially when faced with multiple stimuli. While, in 1987, the world was introduced to RoboCop (Figure 3), there have been few people who would advocate the handling of weapons by a machine. The ethical dilemma of a machine targeting the wrong person is perhaps too large a step to make within the development of AI. While an automated weapon will be programmed with a target mission, the choice of firing a weapon is a complex one, and one that requires an understanding of the threat that a target will cause.

Killer Robots
The growth of autonomous systems within the battlefield has typically scaled to targeted missions. For this, a weapon may be programmed to act autonomously from its controller, and use things such as GPS coordinates and land maps to locate a target. In order to complete the mission, the autonomous system may have to make a decision on the completion of the mission based on what it finds at its target location. For example, an autonomous weapon may find that a group of children is having a party in the region near a building that has been targeted for the dropping of a bomb. The system would then have to weigh up the risks of harming those not directly involved in the mission. The challenge of AI to warfare will thus be a move towards an autonomous system that can fire weapons, especially where it does not put friendly agents and civilians at risk. This will see the rise of UAVs (Unmanned Aerial Vehicles) and which could lead to a term known as ‘killer robots’. Figure 4 shows the Kamikaze Suicide UAV.

These killer robots could be launched underwater, over the air, or even on the ground, and would be smart enough to complete missions of human-like intelligence using vision processing, speech processing, and decision making. It may seem like science-fiction, but a killer robot would be able to travel to a target and then search for a terrorist. They could then locate them in a crowd, and pinpoint their speech patterns, and then assessing the risk to those around the person. These are all decisions that humans must make when assessing that someone is a risk, and then decide on the best course of action.
Unfortunately, humans can get these decisions wrong, such as in the case of Jean Charles de Menezes, who was killed by police who mistook him for a terrorist. Just like a self-driving car, a killer robot would have to have success rates which were much higher than their human equivalents. Ultimately it would likely not take any risks at all if the suspect was surrounded by citizens, and could be trained to only shoot to wound. The cross-over between UAVs and killer robots is a difficult divide, but our society must decide where the boundary stops in what should be allocated, and not. The speed of deployment, and the actual speed of movement, along with the accuracy of the weaponry, would be something that no human army could cope with.
A targeted army of killer robots would likely wipe out any human army in almost an instance. The worry, too, is that an autonomous robot could go wrong. Yul Brynner, in 1973, played a robot in Westworld — the computerized world where nothing could go wrong (Figure 5). Unfortunately, a programming bug caused the robot play by Brynner to go on a killing spree. The film led to the immortal line of “Shut down … shut down immediately!”.
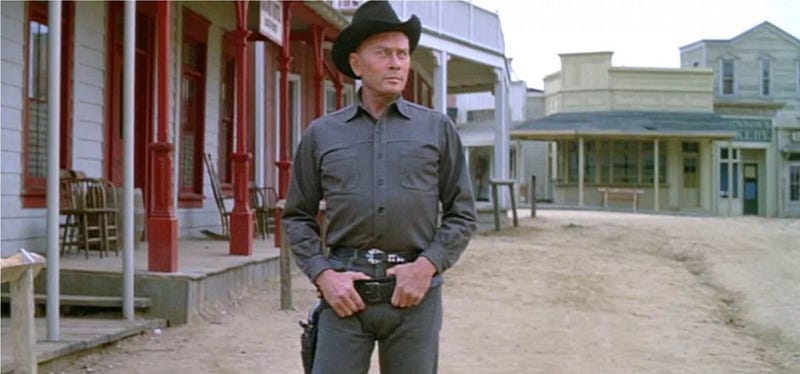
Isaac Asimov, in I, Robot, postulated how future devices will cope with the concept of making a decision on whether a robot should save itself, or a human, with:
- A robot may not injure a human being or, through inaction, allow a human being to come to harm.
- A robot must obey the orders given it by human beings, except where such orders would conflict with the First Law.
- A robot must protect its own existence as long as such protection does not conflict with the First or Second Laws.
He even added a 0th rule which preceded the others to make sure that the rights of society were defined properly:
A robot may not harm humanity, or, by inaction, allow humanity to come to harm
Asimov did not broach the subject of robots making a decision on whether a car which is about to plough into a group of school children should veer off to avoid them, and drive the drivers over a cliff! In the recent adaption of Asimov’s classic novel, the humanization of robots are brought to the fore with the line of “What’s a wink? It’s a sign of trust. It’s a human thing.” (Figure 6).

A hint of a killer robot can be found in Space Odyssey 2001, and where Dr. David Bowman (Dave) and Dr. Frank Poole are pilots on the spaceship controlled by the HAL 9000 computer (HAL). HAL has a human personality and develops a suspicion that the humans have been making mistakes on are thus risking the mission of the ship. Dave and Frank then enter a space pod so that HAL cannot hear them. But HAL is able to lip-read their discussion and determines that the humans aiming to switch him off. HAL then decides that the mission is too important for Dave and Frank to risk it, and casts Frank free from the ship while on a spacewalk. Dave then tries to help Frank, but HAL refuses to allow him back into the space shape. Dave, though, finds a way back in, and HAL ends up pleading with him not to turn him off. HAL’s emotions eventually turn to fear, and where Dave starts to deactivate his higher level intellect circuits while signing “Daisy Bell” (Figure 7).
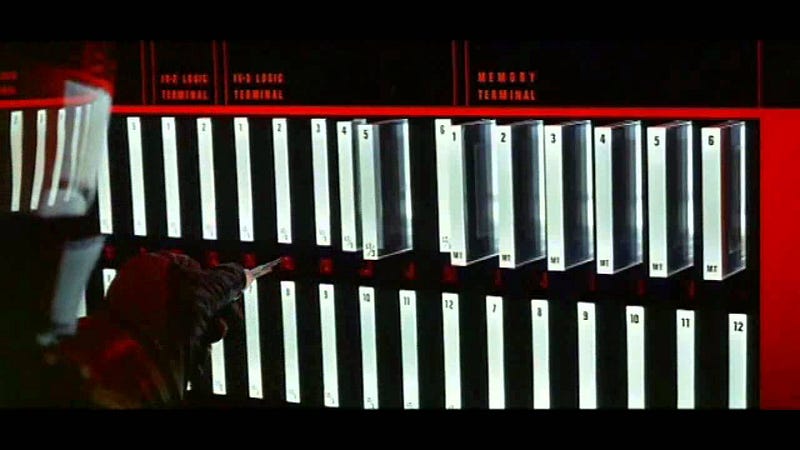
HAL had been programmed to be autonomous and focus on his mission and could learn from his surroundings and make decisions about how best to execute the mission. Thus used all of his superhuman senses to interpret his humans, and determine that they were a threat to the mission. The move to kill the humans just made sense for HAL, as he weighed up the risks against the benefits, and decided that they risked the mission too much and had to be executed. To a computer, humans just seem so error-prone. As humans, we make mistakes, and often driven by bad decisions. We do things that can be seen as being evil. AI systems of the future may be able to make a decision whether humans should be killed, for the sake of a pre-programmed mission.
Perception–cognition–action
At the current time, computer systems are mainly programmed with if-then-else statements and/or a rule-based structure. This allows for a deterministic output, and where we will know how the robot entity will operate. In the following, the robot will scan an area. It will then identify if there is a child present, and not fire its weapon:
objects = scan_objects(area_of_interest);if (determine_objects(objects).Contains(“child”)) {
exit_mission();
} else
{
if determine_objects(objects).Contains(“terrorist”)
{
target=aim_target(location(“terrorist”));
fire_at_target(target);
}
}exit_mission();
The robot, though, can fail to incorrectly detect a child and might go ahead and fire at the terrorist. A fully autonomous system with AI will make its decisions based on probabilities and risk scores, and apply a perception–cognition–action information processing loop. An AI killer robot will thus gather information, then think about it, and finally make a decision based on the inputs and their understanding of the risks involved. Hitchins et al [1] defines this as a SOVA (Sensing, Optimization/Verification and Action) model (Figure 8).
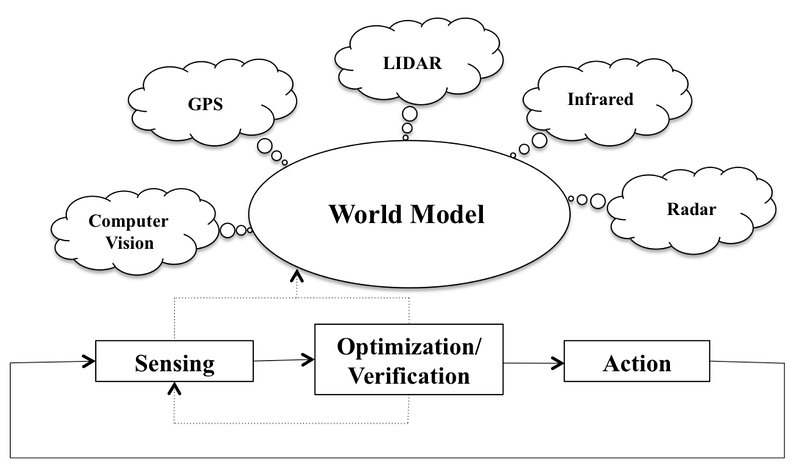
In this case, the SOVA model uses LIDAR, Radar, infrared, GPS and computer vision to create a world model. Hitchens then defines an output quality scale (the left. hand side of Fig 9), and a mission impact scale (the right-hand side of Fig 9). In this case, we define the highest level of accuracy for the sensor information and then map this to the impact scale. If we have Planar (x-y) GPS coordinates we define that the mission will not be adversely affected, but if we only have altitude (z) GPS coordinates, then the mission is likely to fail. This type of approach can be made deterministic and is based on knowledge of the sensing equipment. To rely on just LIDAR Through Glass will mean that the mission will fail.

We can train an autonomous system using Bayesian logic, and where the AI system would learn the probabilities for the success of a mission. But if we apply true cognitive learning — such as with deep learning — an automated machine could actually make a decision for itself as to whether it can trust its own sensors. Over time, and through training, we could define what success involves, and then train the machine to recognise how the sensor information relates to mission success.
Conclusions
Deep learning is coming to our world, and at its scariest, it involves killer robots, and which could replace our existing — and often indiscriminate — bombs. As a society we now see the rise of intelligent cars, and, if you now weaponize these vehicles it is a short hop to killer robots.
Well, here’s my viewpoint:
References
[1] Hutchins, A. R., Cummings, M. L., Draper, M., & Hughes, T. (2015, September). Representing Autonomous Systems’ Self-Confidence through Competency Boundaries. In Proceedings of the Human Factors and Ergonomics Society Annual Meeting (Vol. 59, №1, pp. 279–283). Sage CA: Los Angeles, CA: SAGE Publications. [Link]
[2] Cummings, M. M. (2014). Man versus machine or man+ machine?. IEEE Intelligent Systems, 29(5), 62–69 [Link].